Sleep Cycle REM Detection Accuracy: Wearables vs Bedroom Sensors vs App-Only Tracking
Curious about how your sleep tracker measures REM sleep? We compare the accuracy of wearables, bedroom sensors, and phone apps. The results might surprise you…
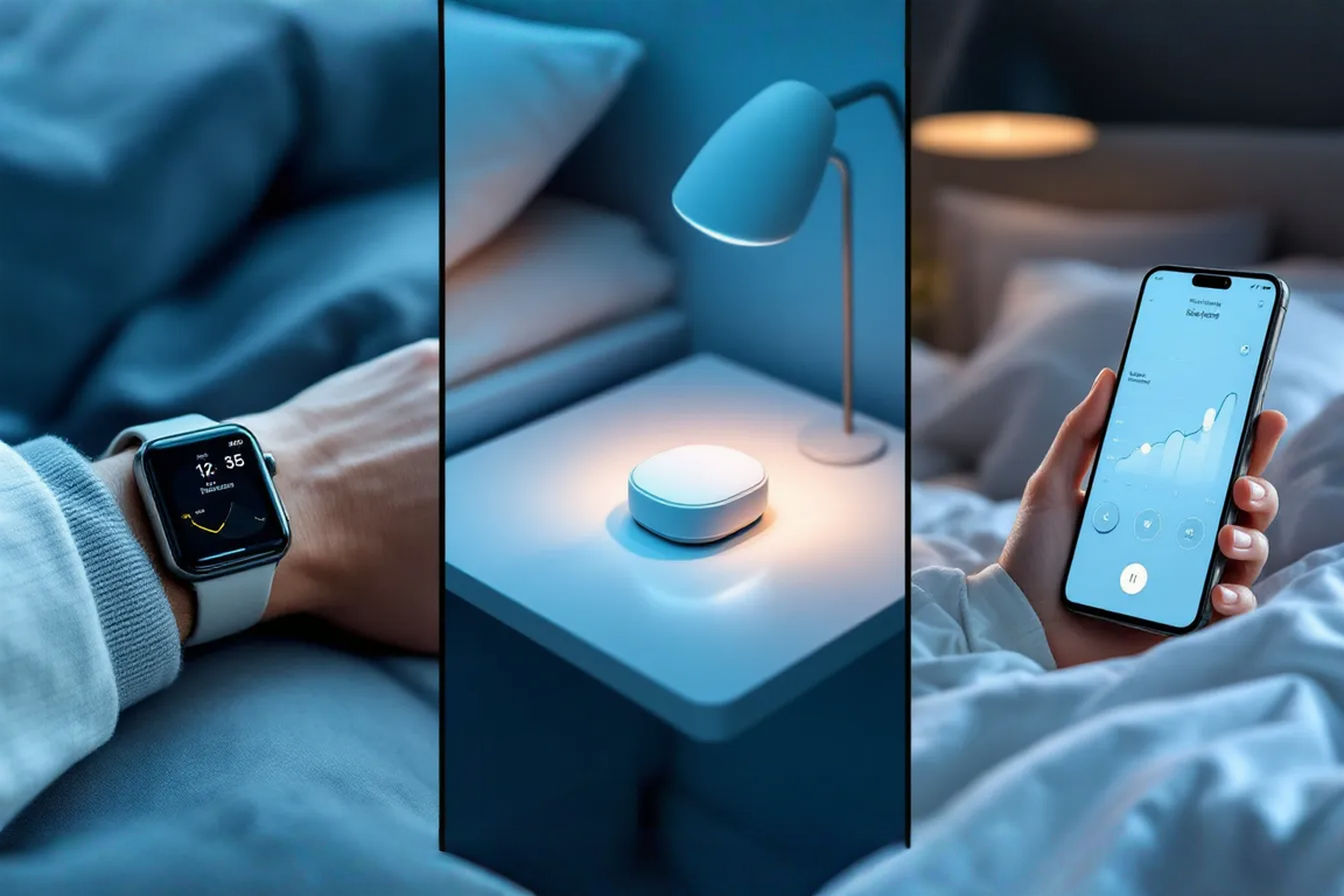
This post may contain affiliate links. If you make a purchase through these links, we may earn a commission at no additional cost to you.
Understanding REM Sleep and Its Importance
Before we talk about tracking it, let’s understand what REM sleep is. Sleep isn’t just one continuous state. It’s a complex process that cycles through different stages throughout the night. These stages are broadly divided into Non-Rapid Eye Movement (NREM) sleep and Rapid Eye Movement (REM) sleep.
NREM sleep has its own stages:
- NREM Stage 1 (N1): This is the lightest stage, the transition from being awake to falling asleep. Your brain waves begin to slow down. It usually only lasts a few minutes.
- NREM Stage 2 (N2): You are now definitely asleep, but still in a relatively light stage. Your heart rate and breathing slow down, and your body temperature drops slightly. Your brain activity shows specific patterns called sleep spindles and K-complexes. This stage makes up a significant portion of your total sleep time.
- NREM Stage 3 (N3): This is often called deep sleep or slow-wave sleep. Your brain waves are very slow (delta waves). It’s the most restorative stage of sleep for your body, important for physical recovery, growth, and immune function. It’s harder to wake someone up during deep sleep.
Then comes REM sleep. This stage is quite different from NREM. Your brain activity during REM sleep looks surprisingly similar to when you’re awake, which is why it’s sometimes called paradoxical sleep. Key characteristics of REM sleep include:
- Rapid Eye Movements: As the name suggests, your eyes dart back and forth quickly under your eyelids.
- Increased Brain Activity: Brain waves speed up and become more variable.
- Muscle Atonia: Your voluntary muscles become temporarily paralyzed. This is thought to prevent you from acting out your dreams.
- Increased Heart Rate and Breathing: Your heart rate and breathing may become faster and more irregular.
- Dreaming: This is when most vivid dreaming occurs.
Why is REM sleep so important? Getting enough REM sleep is crucial for cognitive functions. It’s strongly linked to memory consolidation, particularly for processing emotions and connecting new learning with past experiences. It also plays a role in mood regulation and problem-solving creativity. A lack of sufficient REM sleep can contribute to difficulties with learning, poor concentration, irritability, and even mental health challenges.
A typical night’s sleep involves cycling through these stages multiple times. The cycle usually goes from N1, N2, N3, back to N2, and then into REM. A full cycle takes about 90-110 minutes. Early in the night, you spend more time in deep sleep (N3). As the night progresses, the REM stages get longer, and deep sleep periods shorten.
How Sleep Tracking Technology Works
Consumer sleep trackers don’t use the same methods as a professional sleep lab. The gold standard for sleep stage detection is Polysomnography (PSG). This involves hooking a person up to numerous sensors in a lab setting. PSG measures brain waves (EEG), eye movements (EOG), muscle activity (EMG), heart rate, breathing, and oxygen levels. Trained technicians analyze the data to precisely identify sleep stages minute by minute.
Consumer devices, however, rely on much simpler, non-invasive methods. They primarily use motion and physiological signals to estimate sleep stages. The core idea is that different sleep stages are associated with different patterns of movement, heart rate, breathing, and sometimes sounds.
Here’s a simplified look at the signals used:
- Movement (Actigraphy): This is the most common signal used by trackers. Devices like accelerometers (found in phones, watches, and fitness bands) detect when you move and how much. The assumption is that you move less when you are in deeper sleep stages (N2, N3) and might have specific movement patterns during REM sleep (though the paralysis makes this tricky) or during brief awakenings.
- Heart Rate and Heart Rate Variability (HRV): Many wearables and some bedroom sensors measure your heart rate and the variation in time between heartbeats (HRV). Your heart rate tends to be lower and more stable during deep sleep compared to REM sleep, where it might become more variable.
- Breathing Patterns: Some bedroom sensors, particularly under-mattress or bedside units, can track your breathing rate and rhythm. Breathing patterns can change across sleep stages.
- Sound Analysis: Sleep apps often use the phone’s microphone to listen for sounds like snoring, talking, or overall room noise. While not directly tied to sleep stages, this can help identify disturbances or periods of wakefulness.
The raw data from these sensors is fed into an algorithm. This algorithm is the “brain” of the sleep tracker. It uses complex mathematical models and often machine learning techniques to interpret the sensor data and make an educated guess about which sleep stage you are in at any given time (often in 30-second increments, called epochs, similar to PSG). The algorithm compares the patterns it detects to known patterns associated with sleep stages based on data from studies, though the quality and size of these datasets vary greatly between manufacturers.
It’s crucial to understand that consumer sleep trackers do not directly measure brain waves (EEG), which is the primary signal used by PSG to definitively identify sleep stages, especially REM sleep. This fundamental difference is the main reason why consumer sleep tracking, while convenient, is inherently less accurate than a clinical sleep study. They infer stages based on secondary physiological signals.
Wearable Sleep Trackers: Accuracy on Your Wrist or Finger
Wearable sleep trackers are arguably the most popular type of consumer sleep technology. These include smartwatches, fitness bands, and smart rings. They are worn directly on the body, typically on the wrist or finger.
How They Work and Detect Signals
Wearables primarily rely on two main types of sensors:
- Movement Sensors (Accelerometers and Gyroscopes): These detect motion and orientation. They track how much you move during the night. Algorithms use this data assuming less movement means deeper sleep. While muscle atonia occurs during REM, there might be brief twitches or shifts, or movements during mini-arousals, which the accelerometer picks up.
- Optical Heart Rate Sensors (PPG): These use a technology called photoplethysmography (PPG). Green LED lights are shined through the skin (usually on the wrist or finger), and a photodetector measures the light reflected back. Blood absorbs green light, so changes in light absorption indicate changes in blood flow, which corresponds to your pulse. By tracking pulse over time, the device calculates heart rate. Some advanced wearables also estimate blood oxygen saturation (SpO2) using red and infrared light, although this is less commonly used for basic sleep staging and more for detecting potential breathing issues like sleep apnea.
Wearables attempt to detect REM sleep by analyzing patterns in movement combined with changes in heart rate and heart rate variability (HRV). During REM, heart rate tends to be higher and more variable compared to deep sleep. The algorithm looks for periods of relatively low movement (due to muscle atonia) coupled with increased heart rate and variability, differentiating it from NREM stages.
Accuracy Claims vs. Validation Studies
Manufacturers often market their wearables with claims of high sleep tracking accuracy. However, independent validation studies that compare wearable data to PSG results often show a more nuanced picture, especially for specific sleep stages like REM.
While wearables can be reasonably good at determining if you are awake or asleep (this is called sleep-wake detection, which is largely based on movement), their accuracy in classifying specific sleep stages (NREM stages vs. REM) is generally less precise.
For REM sleep, studies have shown that wearables can sometimes overestimate or underestimate the amount of time spent in this stage. They might confuse periods of light sleep or wakefulness with REM, or miss periods of true REM sleep. The agreement between wearable-detected REM and PSG-detected REM is often lower than the agreement for deep sleep or overall sleep time. Some studies report epoch-by-epoch agreement for sleep stages (including REM) ranging from 50% to 80% compared to PSG, depending on the device and the algorithm used. This means for a given 30-second window, the wearable correctly identifies the stage only 50-80% of the time.
The wrist is a common placement, but it may not always capture the subtle physiological signals as accurately as other body locations or types of sensors. Finger-based wearables, like smart rings, might potentially capture stronger PPG signals due to better contact with arteries, which could theoretically improve heart rate and HRV data, potentially leading to slightly better REM detection, but this is still subject to algorithm limitations.
Pros and Cons for REM Detection
Pros:
- Convenience: Wearables are easy to use and wear nightly.
- Collects Multiple Data Points: Combines movement, heart rate, and sometimes HRV, providing more data than just movement alone.
- Personalized Data: Tracks your sleep over time, allowing you to see trends.
Cons:
- Indirect Measurement: Doesn’t measure brain waves (EEG), which is essential for definitive REM identification.
- Reliance on Movement: Muscle atonia during REM makes movement-based detection challenging.
- Algorithm Dependency: Accuracy heavily relies on the sophistication and training data of the proprietary algorithm. Different devices from different companies will have varying levels of accuracy.
- Skin Contact Issues: Poor fit or movement can affect the accuracy of heart rate readings.
In short, while wearables provide a convenient estimate of your sleep stages, including REM, their accuracy for this specific stage is limited compared to a clinical study. They can show you patterns and trends in your estimated REM sleep over time, which can be useful, but the exact minutes recorded each night should be viewed as approximations rather than precise measurements.
Bedroom Sleep Sensors: Non-Contact Accuracy
Bedroom sleep sensors offer a different approach by tracking your sleep without requiring you to wear anything. These devices are typically placed under your mattress or on your bedside table.
How They Work and Detect Signals
Bedroom sensors use various technologies to monitor your physiological signals remotely:
- Ballistic Cardiography (BCG) / Seismocardiography (SCG): These sensors, often placed under the mattress, are incredibly sensitive to the micro-vibrations caused by your body’s functions, primarily your heartbeat and breathing. Every heartbeat and breath causes tiny movements and vibrations that travel through your body and the mattress. BCG/SCG sensors measure these subtle movements. From this vibration data, the sensor can derive heart rate, heart rate variability, and breathing rate and patterns.
- Simplified: Imagine a super-sensitive microphone picking up the tiny shakes inside your body from your heart beating and lungs breathing, through the mattress.
- More Detailed: BCG specifically focuses on the whole-body recoil from blood ejection from the heart, while SCG measures vibrations on the chest surface. Under-mattress sensors often capture a combination of these signals. Piezoelectric sensors or specialized accelerometers are used to detect these minute mechanical waves.
- Radar or Ultra-Wideband (UWB): Some bedside sensors use radar technology. They emit low-power radio waves towards your body and measure the reflected waves. The slight movements of your chest as you breathe or your body shifts cause changes in the reflected signal’s frequency or phase (the Doppler effect). This allows the sensor to track breathing rate, depth, and heart rate non-contact.
- Simplified: Like a tiny radar gun pointed at your chest that can see it move as you breathe and your heart beats.
- More Detailed: These systems typically use continuous wave or pulsed radar in a specific frequency band. Signal processing algorithms analyze the received signal’s modulation caused by physiological movements.
- Infrared Sensors: Some bedside sensors might use passive infrared (PIR) sensors to detect body heat and movement, though these are generally less sophisticated for detailed sleep staging compared to BCG or radar, often just indicating presence or large movements. More advanced infrared sensors might use thermal imaging to detect breathing-related temperature changes.
Bedroom sensors attempt to detect REM sleep by analyzing patterns in breathing, heart rate, and micro-movements. During REM, breathing can become shallower and more irregular, heart rate increases and is more variable, and while large muscle movement is suppressed, there might be subtle twitches or changes in position detected by the sensitive BCG/SCG or radar systems. The algorithms look for the combination of these signals that corresponds to known REM patterns.
Accuracy Claims vs. Validation Studies
Bedroom sensors, particularly those using BCG or radar, have the potential to capture more direct physiological signals (breathing and heartbeat mechanics) than just limb movement and peripheral heart rate from a wrist. This can lead to potentially better accuracy in differentiating sleep stages, including REM, compared to basic movement-only trackers.
Validation studies comparing BCG or radar-based sensors to PSG have shown promising results. Some studies indicate that these sensors can achieve better agreement with PSG for sleep staging, including REM, than many wearable devices. Accuracy can vary, but some high-quality sensors report epoch-by-epoch agreement in the 80%+ range for overall sleep staging, with REM detection being a key component of that.
However, accuracy can still be affected by factors like:
- Mattress Type: Very thick or soft mattresses can sometimes dampen the micro-vibrations, affecting BCG/SCG sensor performance.
- Multiple Occupants: If the sensor is under a shared mattress, it can pick up signals from both individuals, complicating the data analysis unless specifically designed to separate them.
- Placement: The exact positioning of the sensor under the mattress or the bedside unit’s angle can impact performance.
- Algorithm Quality: Just like with wearables, the sophistication of the algorithm that interprets the raw sensor data is crucial.
Pros and Cons for REM Detection
Pros:
- Non-Contact: No need to wear anything, which is preferred by many.
- Captures Direct Physiological Signals: BCG and radar can directly measure breathing and heart mechanics, which are key indicators of sleep stages.
- Potentially Higher Accuracy: Some studies suggest better sleep staging accuracy, including for REM, compared to many wearables, especially those relying only on movement.
Cons:
- Less Portable: Stays in the bedroom, not useful for tracking sleep while traveling unless you bring the device.
- Installation/Setup: May require specific placement under the mattress or on a table.
- Potential Interference: Multiple people in the bed or certain environmental factors can sometimes affect readings.
- Still Not PSG: While potentially more accurate than some wearables, they still don’t measure brain waves (EEG), so they remain estimates.
Bedroom sensors offer a compelling alternative with the potential for improved REM detection accuracy by directly measuring physiological signals like breathing and heartbeat dynamics without requiring user interaction during sleep.
App-Only Sleep Tracking: Relying on Your Phone’s Sensors
The simplest and often cheapest way to track sleep is by using an app on your smartphone. These apps leverage the sensors already built into your phone.
How They Work and Detect Signals
App-only sleep trackers primarily use two of your phone’s sensors:
- Accelerometer: Your phone has an accelerometer to detect its movement and orientation. If you place your phone on your mattress (often instructed to place it near your pillow or under a corner of the mattress), the app uses the accelerometer to track the vibrations caused by your body movements throughout the night. The assumption is that less movement means deeper sleep.
- Microphone: The app can use the phone’s microphone to listen to sounds while you sleep. This data is primarily used to detect snoring, sleep talking, or environmental noises that might disturb your sleep. Some apps might attempt to correlate periods of silence or specific sound patterns with sleep stages, but this is less common and highly unreliable for staging.
App-only trackers attempt to detect REM sleep almost entirely based on movement patterns picked up by the accelerometer. The algorithms look for periods of relative stillness (potentially indicating deep sleep or REM’s muscle atonia) interspersed with periods of greater movement (indicating lighter sleep, wakefulness, or potentially the subtle movements or mini-arousals within REM). Sound data might be used to flag periods of wakefulness triggered by noise or snoring.
Accuracy Limitations
The accuracy of app-only sleep tracking for REM detection is generally considered the lowest among the three categories. This is due to several significant limitations:
- Limited Sensor Data: The phone only has accelerometers and microphones readily available for sleep tracking. It lacks the physiological sensors found in wearables (PPG for heart rate) or bedroom sensors (BCG/radar for heart/breathing mechanics). Movement alone is a poor proxy for differentiating between sleep stages, especially between light sleep, REM, and even brief periods of wakefulness where you aren’t moving much.
- Placement Sensitivity: The accuracy is highly dependent on where you place your phone. If it’s too far away, it won’t pick up your movements. If it’s on a soft surface or moves during the night, the data can be inaccurate.
- External Factors: The accelerometer can pick up vibrations from external sources, like someone else getting into or out of bed, a pet jumping on the bed, or even vibrations from outside the room, which can be misinterpreted as your movement. The microphone can pick up noise from outside your bedroom, affecting sound analysis.
- Basic Algorithms: Many free or low-cost sleep apps use relatively simple algorithms compared to the sophisticated ones developed by companies specializing in sleep technology.
Because app-only tracking relies so heavily on movement and has limited physiological data, distinguishing REM sleep from other stages is very difficult. The muscle paralysis in REM means there’s little movement, which could be misinterpreted as deep sleep (N3) or even light sleep (N2). The subtle physiological changes characteristic of REM (heart rate, breathing) are not directly measured.
Pros and Cons for REM Detection
Pros:
- Cost-Effective: Often free or a low one-time cost as it uses existing hardware.
- Convenient: Uses a device you already have.
Cons:
- Lowest Accuracy: Generally the least accurate method for sleep staging, particularly for distinguishing REM from other stages.
- Highly Dependent on Placement: Results can vary wildly based on where the phone is placed on the bed.
- Limited Data: Relies almost entirely on movement and sound, missing crucial physiological signals.
- Battery Drain: Running tracking all night can drain your phone’s battery.
- Potential for Interruption: Notifications or phone calls could potentially disrupt tracking or your sleep.
While app-only trackers can give you a basic idea of your total sleep time and when you might have been restless, they are not reliable for accurate REM sleep detection or precise sleep staging. They are best used for getting a general sense of sleep patterns and identifying major disturbances like snoring.
Comparing REM Detection Accuracy: Wearables vs. Sensors vs. Apps
When it comes to accurately detecting REM sleep, there’s a clear hierarchy based on the types of sensors used and the sophistication of the algorithms.
Here’s a comparison of the typical accuracy for REM detection, relative to the gold standard of PSG:
- Polysomnography (PSG): The gold standard. Utilizes EEG, EOG, EMG, etc., analyzed by trained professionals. Highly accurate for identifying REM sleep based on brain wave patterns, eye movements, and muscle tone. This is the benchmark against which all other methods are compared.
- Bedroom Sensors (BCG/Radar): Generally offer the highest potential accuracy among consumer devices for REM detection. By directly measuring breathing patterns and heart mechanics (HRV, rate changes) through sensitive non-contact sensors, they capture key physiological signatures associated with REM sleep that movement-only methods miss. While not measuring brain waves, these physiological signals are stronger indicators of sleep stages than just body motion.
- Wearable Trackers (PPG + Accelerometer): Offer moderate accuracy for REM detection. They combine movement data with heart rate and HRV. The inclusion of cardiac data improves upon movement-only tracking. Algorithms use the combination of low movement and increased heart rate/variability to estimate REM. Accuracy varies significantly between devices and brands based on sensor quality and algorithm sophistication. Generally less accurate than high-quality bedroom sensors but more accurate than app-only tracking.
- App-Only Trackers (Accelerometer + Microphone): Offer the lowest accuracy for REM detection. Relying almost exclusively on movement patterns from a phone placed on a mattress is a very poor substitute for physiological or brain activity measurement. They struggle significantly to differentiate REM from other stages, particularly light sleep or periods of quiet wakefulness.
It’s important to emphasize that “accuracy” in consumer sleep tracking is often reported in different ways. Some companies might cite the percentage of time the device correctly identifies sleep vs. wake (sleep-wake accuracy), which is usually quite high. However, sleep stage accuracy, especially for specific stages like REM, is much harder to achieve. Validation studies often look at epoch-by-epoch agreement (correctly classifying a 30-second period) or the total time spent in each stage compared to PSG. An epoch-by-epoch agreement for REM of 70-80% compared to PSG would be considered quite good for a consumer device, but it still means the device is incorrect 20-30% of the time for that specific stage.
Furthermore, consumer sleep trackers are generally better at identifying NREM sleep stages (especially distinguishing deep sleep due to its distinct movement and heart rate patterns) than they are at pinpointing the nuanced characteristics of REM sleep.
Validation Methods
The credibility of any sleep tracker’s accuracy claims depends heavily on whether it has been validated against PSG in independent research studies. Look for validation studies published in peer-reviewed scientific journals. These studies compare the device’s output to simultaneous PSG recordings from the same individual. A device that has undergone rigorous, independent validation is more likely to provide reasonably reliable data than one whose accuracy claims are only based on internal testing or anecdotal evidence.
The Gold Standard: Polysomnography (PSG)
To truly understand the limitations of consumer sleep tracking, it’s essential to know what the gold standard, Polysomnography (PSG), involves. PSG is a comprehensive study conducted in a sleep lab or sometimes in a home setting with specialized equipment and technician setup.
During a PSG, a person is connected to multiple sensors that measure:
- Electroencephalogram (EEG): Records brain wave activity. This is the most critical measurement for distinguishing sleep stages, as each stage has distinct electrical patterns in the brain. REM sleep has brain waves similar to wakefulness (low voltage, mixed frequency), which is why it’s called paradoxical.
- Electrooculogram (EOG): Records eye movements. Rapid eye movements are the defining characteristic of REM sleep.
- Electromyogram (EMG): Records muscle activity, typically from the chin and legs. Muscle tone decreases significantly during NREM sleep and is almost completely lost during REM sleep (muscle atonia).
- Electrocardiogram (ECG): Records heart rate and rhythm.
- Respiratory Sensors: Measure airflow through the nose and mouth, chest and abdominal movement (effort), and oxygen saturation levels (SpO2). These help assess breathing patterns and identify sleep-disordered breathing events.
Trained sleep technologists analyze the data collected throughout the night, typically in 30-second epochs. By examining the combination of brain waves, eye movements, and muscle tone, they can accurately identify the beginning and end of each sleep stage, including REM sleep, with high precision.
Consumer sleep trackers, lacking EEG and EOG sensors, cannot directly observe the defining characteristics of REM sleep (brain activity and rapid eye movements). They can only infer the likelihood of REM based on secondary signals like heart rate patterns, breathing changes (if measured), and the absence of large body movements. This is why even the most advanced consumer devices cannot match the accuracy of a PSG for sleep staging.
Factors Affecting Consumer Sleep Tracker Accuracy
Beyond the core technology (wearable vs. sensor vs. app), several other factors can influence the accuracy of REM sleep detection in consumer devices:
- Algorithm Sophistication: This is perhaps the biggest factor. How well is the algorithm designed to interpret the noisy, indirect sensor data? Was it trained on a diverse and large dataset of PSG-validated sleep? Proprietary algorithms vary widely in quality. Companies often update their algorithms, which can potentially improve accuracy over time.
- Individual Differences: People’s physiological signals and sleep patterns vary. Factors like age, sex, body composition, and underlying health conditions can affect heart rate, breathing, and movement patterns during sleep. What the algorithm identifies as REM for one person might be different for another.
- Sleep Disorders: Conditions like sleep apnea (which causes breathing pauses) or Restless Legs Syndrome (which causes leg movements) can significantly disrupt normal sleep architecture and physiological patterns. This can confuse sleep tracking algorithms, leading to inaccurate staging, including misclassification of REM.
- Device Fit and Placement: For wearables, a loose fit can lead to poor heart rate data. For bedroom sensors, incorrect placement can affect the quality of the signal captured. For apps, phone placement is critical.
- Environmental Factors: Room temperature, noise levels, and light can affect sleep patterns and potentially interfere with sensor readings (e.g., noise affecting app microphones).
- Data Artifacts: Sensor data can be affected by glitches or non-sleep-related activities (e.g., getting up to use the restroom). Algorithms need to be robust enough to handle these “artifacts.”
- Time in Bed vs. Time Asleep: Many trackers initiate sleep tracking simply when you are still in bed. However, you might be awake but lying still. This can affect the calculation of total sleep time and the proportion of time spent in each stage if the algorithm doesn’t accurately detect wakefulness within the sleep period.
Considering these factors, even a device with generally good accuracy in validation studies might produce less accurate results for an individual user on a given night.
Choosing the Right Sleep Tracker for Your Needs
Given the differences in accuracy, how do you choose the right sleep tracker? It depends on your goals:
- For a General Overview and Trends: If you want to get a basic idea of your sleep patterns, monitor consistency, see approximate total sleep time, and identify potential disturbances like snoring (using an app), a simple app-only tracker or a basic wearable might suffice. These are useful for motivating better sleep habits by making you more aware of your sleep schedule. However, do not rely on them for accurate sleep stage data, especially REM.
- For More Detailed Tracking and Better REM Estimation: If you are looking for more reliable estimates of your time in different sleep stages, including REM, and are interested in seeing how lifestyle factors (like exercise or caffeine) might affect your sleep architecture, a wearable with good heart rate tracking (like a smartwatch or ring) or, preferably, a bedroom sensor (BCG or radar) validated against PSG would be better choices. Bedroom sensors, by capturing more direct physiological signals, often provide a more nuanced picture of sleep stages than wearables relying primarily on wrist movement and PPG.
- For Clinical Diagnosis or High-Precision Data: If you suspect a sleep disorder (like sleep apnea or narcolepsy, which affects REM) or need truly accurate sleep stage data for health monitoring, you must consult a doctor and likely undergo a Polysomnography (PSG) study in a sleep lab. No consumer device can replace a clinical diagnosis or the precision of PSG.
Think of consumer sleep trackers as helpful tools for gaining insight into your sleep patterns and trends, rather than medical devices providing definitive diagnoses or perfectly precise measurements of each sleep stage. The data, especially for REM sleep, should be interpreted with this understanding.
Limitations and Future of Sleep Tracking Technology
Despite rapid advancements, consumer sleep tracking technology still has significant limitations, particularly regarding REM detection:
- Lack of EEG: The inability to measure brain waves remains the fundamental barrier to achieving PSG-level accuracy for sleep staging.
- Inferential vs. Direct Measurement: Consumer devices infer sleep stages from secondary signals rather than directly measuring the electrical activity of the brain that defines those stages.
- Algorithm Opacity: The proprietary nature of most algorithms makes it difficult for users and researchers to fully understand how the device arrives at its conclusions or troubleshoot inaccuracies.
- Focus on Average User: Algorithms are typically trained on data from a general population, which may not accurately reflect the unique sleep physiology of individuals, especially those with atypical sleep patterns or disorders.
However, the field is constantly evolving. Future advancements might include:
- Integration of More Sensor Types: Combining data from multiple types of sensors (e.g., a wearable wrist sensor with a bedside radar) could improve accuracy.
- Improved Algorithms: As machine learning techniques become more sophisticated and trained on larger, more diverse datasets, algorithms will get better at recognizing patterns associated with different sleep stages from indirect signals.
- Development of Simpler EEG Solutions: Research is ongoing into less intrusive methods of measuring brain activity outside of a full sleep lab, though bringing this to mass-market consumer devices is challenging.
- Personalized Algorithms: Future devices might use longer-term personal data to tailor their algorithms to an individual’s unique physiological patterns, potentially improving accuracy over time for that user.
While a consumer device achieving PSG-level REM detection accuracy is unlikely in the near future without significant technological shifts, the technology will likely continue to improve, offering increasingly valuable insights into sleep patterns and contributing to better sleep health awareness.
Conclusion
REM sleep is a vital part of a healthy sleep cycle, essential for cognitive function, memory, and mood. The rise of consumer sleep tracking technology—from wearables and bedroom sensors to app-only solutions—has made it easier than ever to monitor sleep at home. However, the accuracy of these devices in detecting specific sleep stages, particularly REM, varies significantly.
App-only trackers, relying primarily on smartphone movement sensors, offer the lowest accuracy for REM detection due to limited data input. Wearables, combining movement with heart rate data, provide a better estimate but are still limited by the indirect nature of their measurements compared to brain activity. Bedroom sensors using technologies like BCG or radar show the most promise among consumer devices for more accurate REM detection by capturing more direct physiological signals like breathing and heart mechanics.
Ultimately, no consumer sleep tracker can match the precision of Polysomnography (PSG), the clinical gold standard that measures brain waves directly. Consumer devices infer sleep stages based on secondary signals and algorithms.
If you’re using a sleep tracker to get a general idea of your sleep habits and notice trends, any of these might offer some value. However, if you’re seeking more reliable data on your time spent in REM sleep to understand potential impacts on your cognitive function or to discuss with a healthcare professional, a bedroom sensor validated against PSG is likely your best consumer option. Always remember that these tools are for insights into patterns, not for diagnosing sleep disorders. For true diagnostic accuracy regarding your sleep architecture, consult a medical professional and consider a clinical sleep study. Understanding the limitations of your device is key to interpreting your sleep data effectively and using it to support your overall health.